Agro-industrial complex
Innovations in agriculture
The intensive growth of agricultural production and the increase in the efficiency of crop farming, livestock farming, and the processing of agricultural products are driven by the adoption of advanced technologies across related industries, including:
- Mechanical engineering and automation
- Breeding and genetics
- Feed and fertilizer production
High competition and the demand for increased productivity are accelerating the digitalization of agricultural production in both small farms and large agro-industrial complexes. Advanced AI technologies enhance efficiency, reduce environmental impacts, improve forecasting, optimize costs, and ensure effective monitoring and control of production processes.
AI in agriculture
Proven AI applications in agriculture
Full automation of planting and harvesting machinery
Unmanned monitoring of crop fields and livestock grazing areas
Predictive cost and profit analytics through enterprise management automation
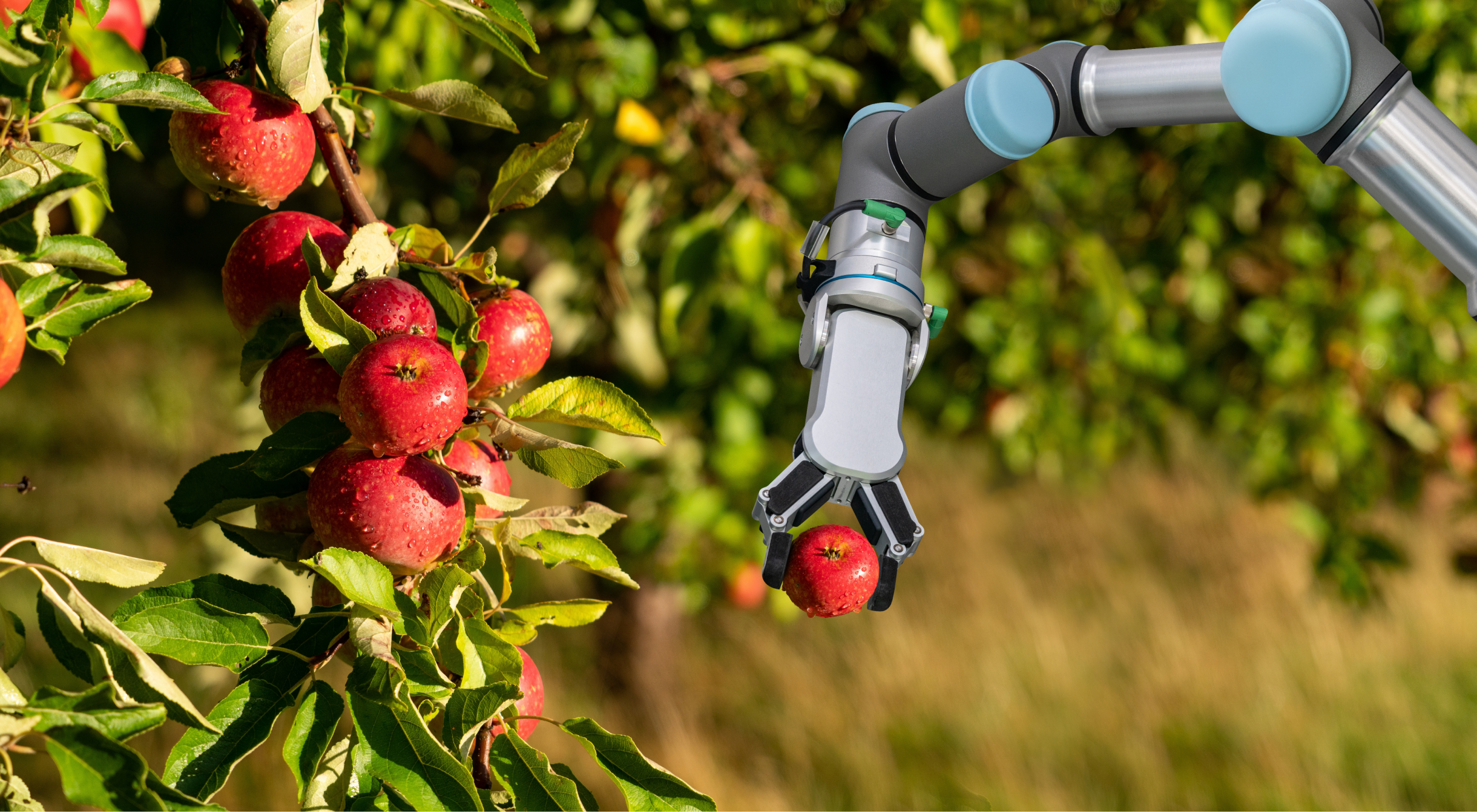
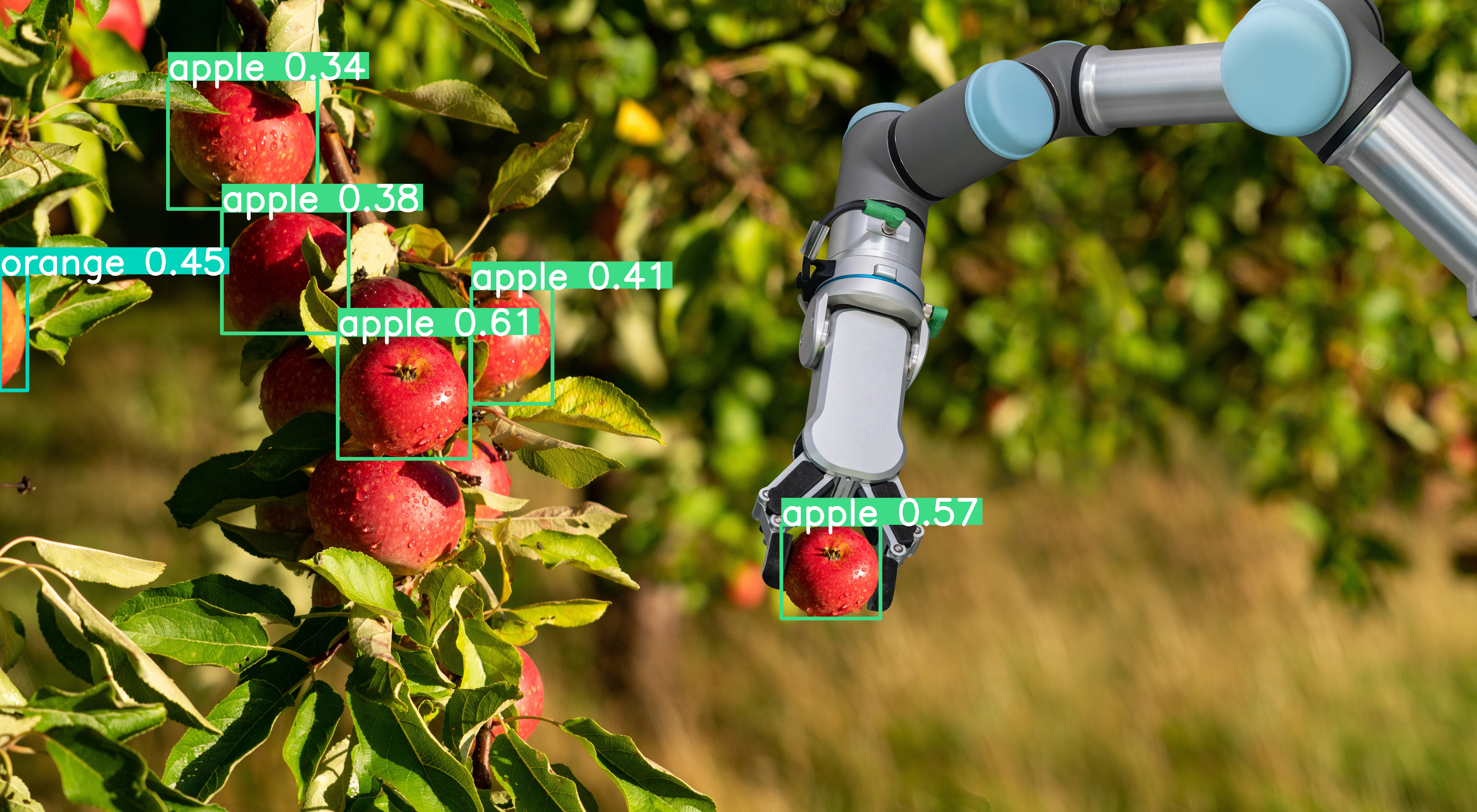
AI threats in agriculture
AI introduces benefits but also risks, including in agriculture. While the security of AI systems in agricultural machinery and production is typically the responsibility of system developers, the security of AI systems for enterprise management and control rests on the farms and complexes themselves. This is primarily because the data for these AI systems is generated directly on-site, making it unique, specific, and impossible to import from external sources. This is also where the key vulnerabilities for such AI systems lie:
- The quality and security of the data used
- The protection of AI models built on this data
Below we review these risks and provide recommendations to mitigate them.
Data
Data here refers to the knowledge base that usually encompasses all an enterprise’s lifecycles, including around farming conditions and methods, successes and failings, making it highly sensitive and confidential. Data leakage - including through unreliable AI systems – is the primary risk. Threats include:
- Malicious backdoors embedded in AI models
- Data storage within an AI model’s weights
Protection against these vulnerabilities includes only using AI models from trusted providers. Downloading models from unverified online sources carries significant risks!
Example of an AI model attack: object misclassification
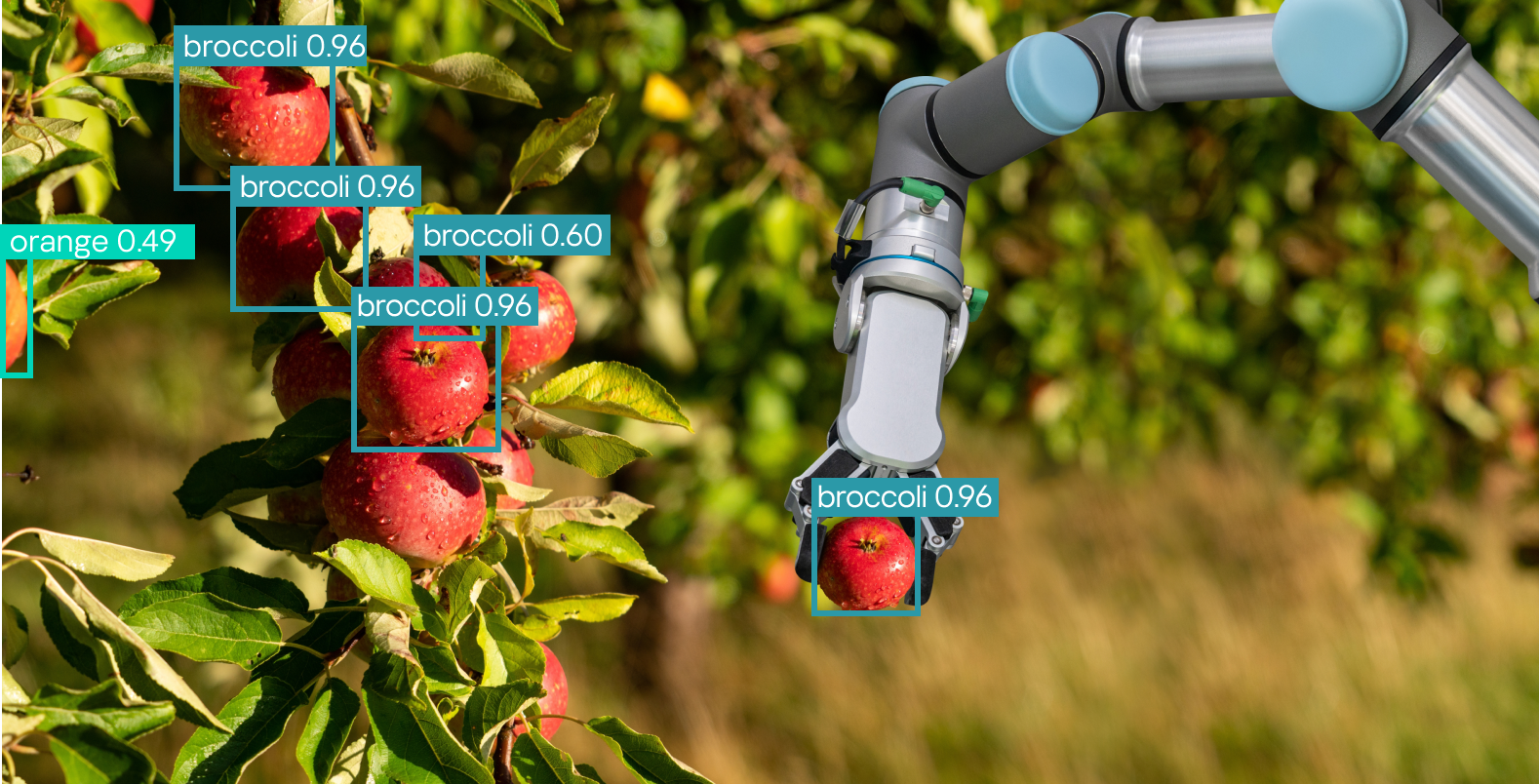
AI Model
The training data used to build AI models plays a crucial role. Factors such as correctness, completeness, impartiality and others [5, 6, 7] directly influence the quality and reliability of the resulting model. Training an AI model involves assimilating training data to reproduce learned behavior. And here lies another danger: the illegitimate copying (replication) of the model, along with the expertise it contains. Such copying can occur even without direct access to the model, simply by manipulating its inputs and outputs.
Model protection
The effectiveness of model copying depends on factors such as understanding the model's internal structure, the level of access to it, and so on [8, 9]. Protective measures include a range of methods for encrypting input and output information, integrating tracking modules to detect copying attempts, and others.
Conclusion
As the range of applied AI technologies continues to grow, additional vulnerabilities are likely to emerge. These are explored in other sections of this website dedicated to agriculture and related industries.
If you have any questions, please contact us: aist@kaspersky.com
References
Expand
- 1. GOST R 59920-2021 «Artificial intelligence systems. Artificial intelligence systems in agriculture. Requirements for provisioning the operating safety of autonomous driving systems for agricultural machines».
- 2. PNST 868-2023 «Artificial intelligence in plant growing. Use cases for process control automation».
- 3. PNST 869-2023 «Artificial intelligence in animal husbandry. Use cases for process control automation».
- 4. PNST 870-2023 «Artificial intelligence in the processing of agricultural products and food production. Use cases for process control automation».
- 5. ETSI GR SAI 002 V1.1.1 (2021-08) «Securing Artificial Intelligence (SAI); Data Supply Chain Security», NEQ
- 6. ITU-T/SG 13 «Big data - Overview and requirements for data preservation».
- 7. ISO/IEC TR 24027:2021 «Information technology — Artificial intelligence (AI) — Bias in AI systems and AI aided decision making».
- 8. Tramèr F. et al. Stealing machine learning models via prediction {APIs} //25th USENIX security symposium (USENIX Security 16). – 2016. – С. 601-618.
- 9. Jagielski M. et al. High accuracy and high fidelity extraction of neural networks //29th USENIX security symposium (USENIX Security 20). – 2020. – С. 1345-1362.